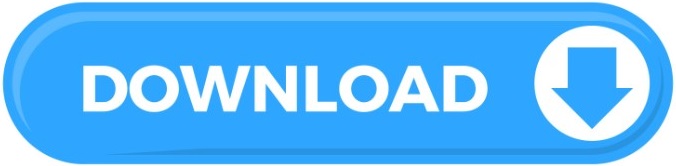
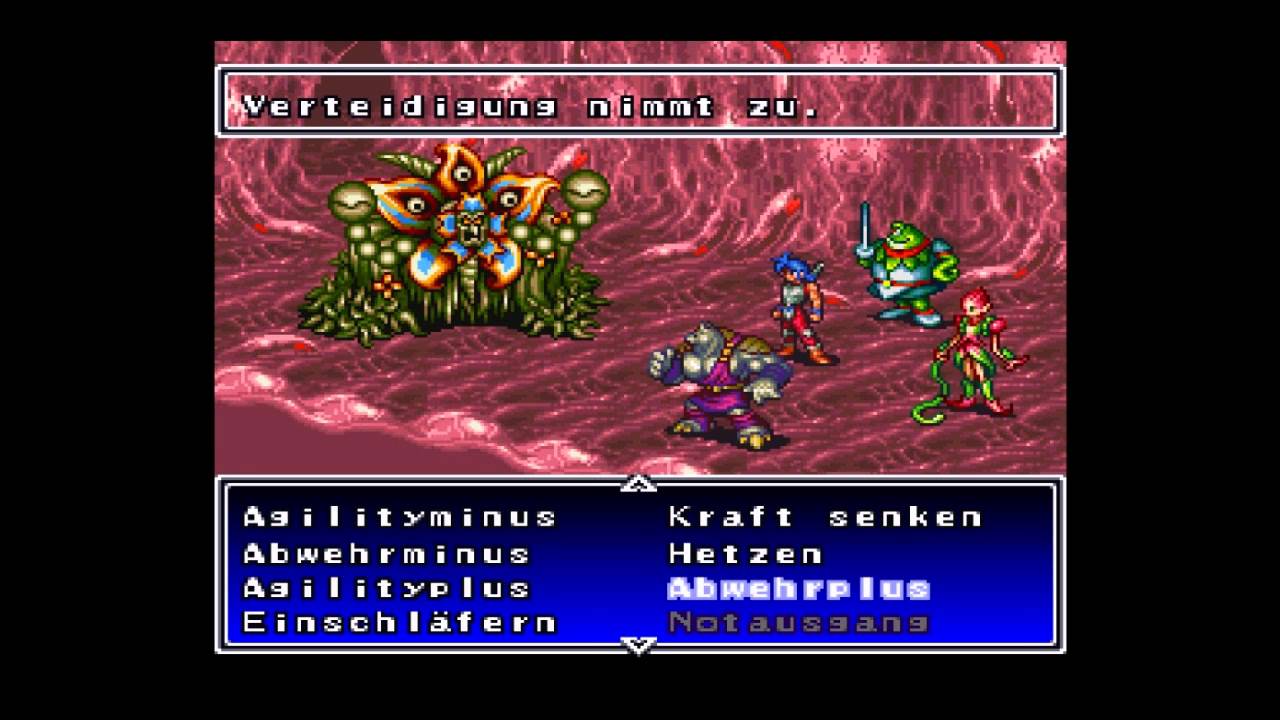
The list of possible choices can be pre-trained ( fit) to accelerate the computation in The main difference is that the Levenshtein distance is replaced by the Joint Complexity distance. The fuzz module mimicks the fuzzywuzzy-like packages like

It is in fact very similar to sklearn’s CountVectorizer using char orĬhar_wb analyzer option from that module. Its scikit-learn counterpart (also named CountVectorizer). The main entry point for this module is the CountVectorizer class, which mimicks It is possible to fit only a random sample of factors to reduce space and computation time. The features can be incrementally updated With a focus on character-based extraction. The feature_extraction module mimicks the module Bag of Factors allow you to analyze a corpus from its factors.įree software: GNU General Public License v3
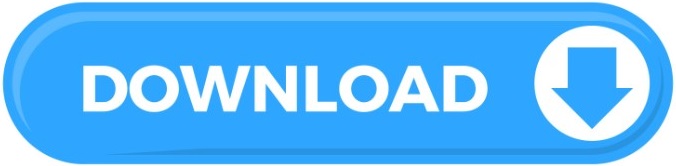